What is machine learning and how it works
Machine learning (ML) is the use of mathematical data models that help a computer learn without direct instructions. It is considered a form of artificial intelligence (AI). Machine learning uses algorithms to identify patterns in data. Based on these patterns, a data model is created for prediction. The more data such a model processes and the longer it is used, the more accurate the results become. This is very similar to how humans hone skills in practice.
Because of the adaptive nature of machine learning, it is great for scenarios where the data is constantly changing, the properties of queries or problems are unstable, or it is virtually impossible to write code to solve.
Useful Articles
From The Blog
-
Unveiling the Limits: Why AI Hasn’t Fully Replaced Web Developers…Yet
By
Welcome to a world of endless possibilities, a world where advancements in Artificial Intelligence (AI) have transformed the way we live and work. From self-driving cars to intelligent personal assistants, AI’s impact on various industries is undeniable, revolutionizing how we approach tasks and processes that were once exclusively human domain. In the realm of web […]
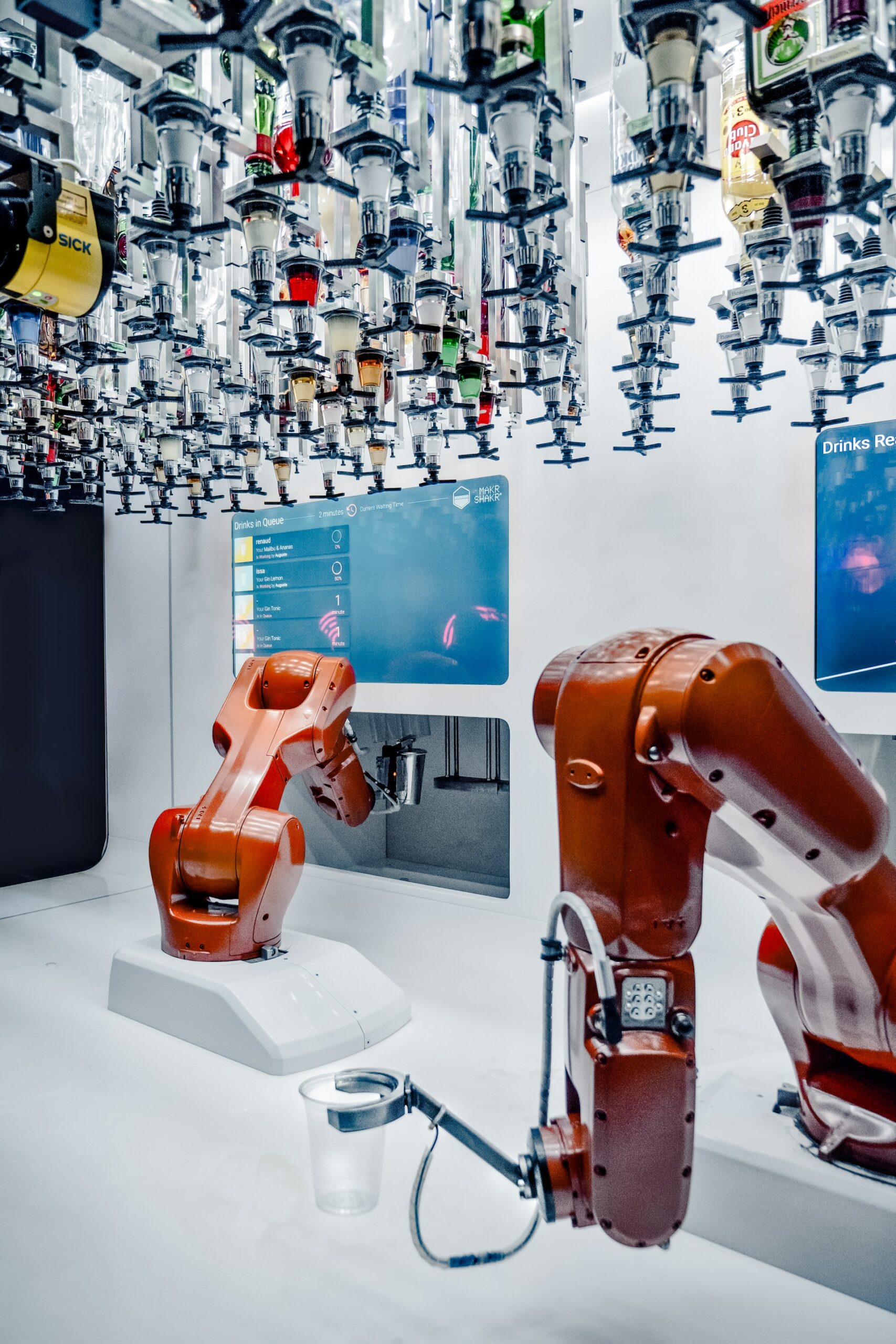
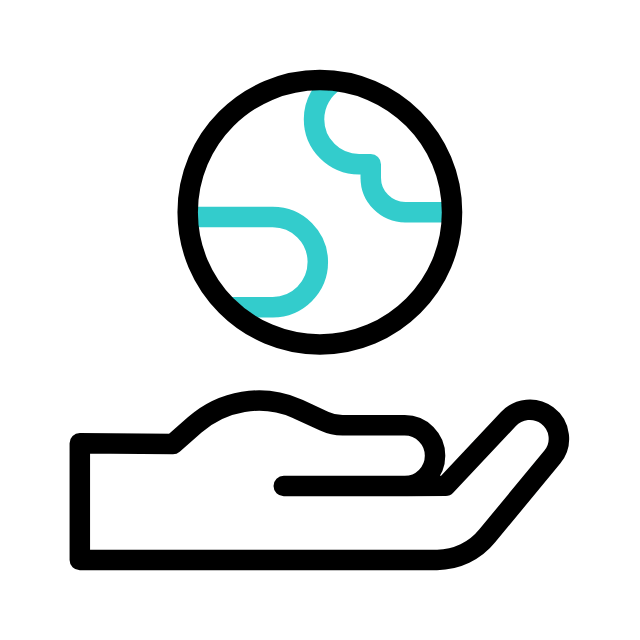
2k+ industries use machine learning
AI In Life
Using machine learning in different industries
Enterprises in a number of industries use machine learning in a variety of ways. The following are examples of applications of machine learning in major industries.
- Banking and Finance
- Transportation
- Agriculture
- Health Care
Company use machine learning
Trusted & Partnered With…
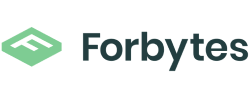
Forbytes, an engineering software company, is a top provider of bespoke tech solutions for businesses across the globe. We are passionate about what we do and ready to share our extensive experience.
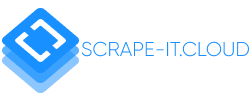
Explore the potential of machine learning applications with Scrape-It.Cloud, a reliable web scraping API.
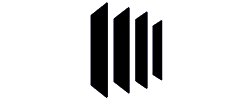
Embark on a design adventure at The Designest. Discover captivating visuals, inspiring resources, and unleash your creativity with the mesmerizing text effect for daring and adventurous designs.
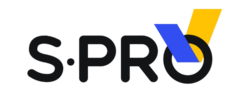
S-PRO is a leading mobile app development company, specializing in delivering innovative and user-centric solutions to businesses worldwide.
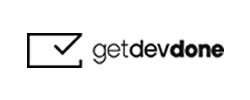
GetDevDone is a white-label WordPress agency partnering with various digital and marketing agencies for more than 15 years. Get all your WordPress needs covered with GetDevDone.
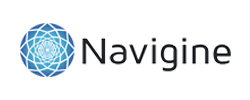
Looking for an efficient way to manage your assets? Bluetooth location tracking technology can help you locate your assets quickly and easily, saving you time and money.
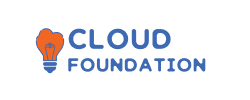
NetSuite experts have created the course curriculum with a use-case oriented and practical approach to provide students hands-on experience learning about the platform.
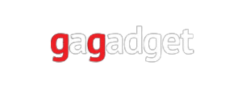
Find the best conference room camera for your needs right here at https://gagadget.com/
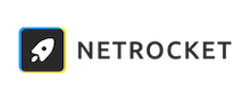
Netrocket offers high-quality SaaS SEO services to enhance your online presence and drive growth
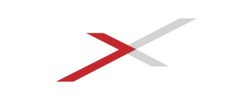
Oxagile, a team of expert AI software developers, excels in delivering groundbreaking AI-driven solutions, pushing the boundaries of technological advancement and driving business success.
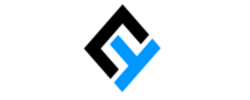
Delve into the world of IoT with expert insights by Cogniteq, highlighting the top companies that are at the cutting edge of technological advancement and industry evolution.
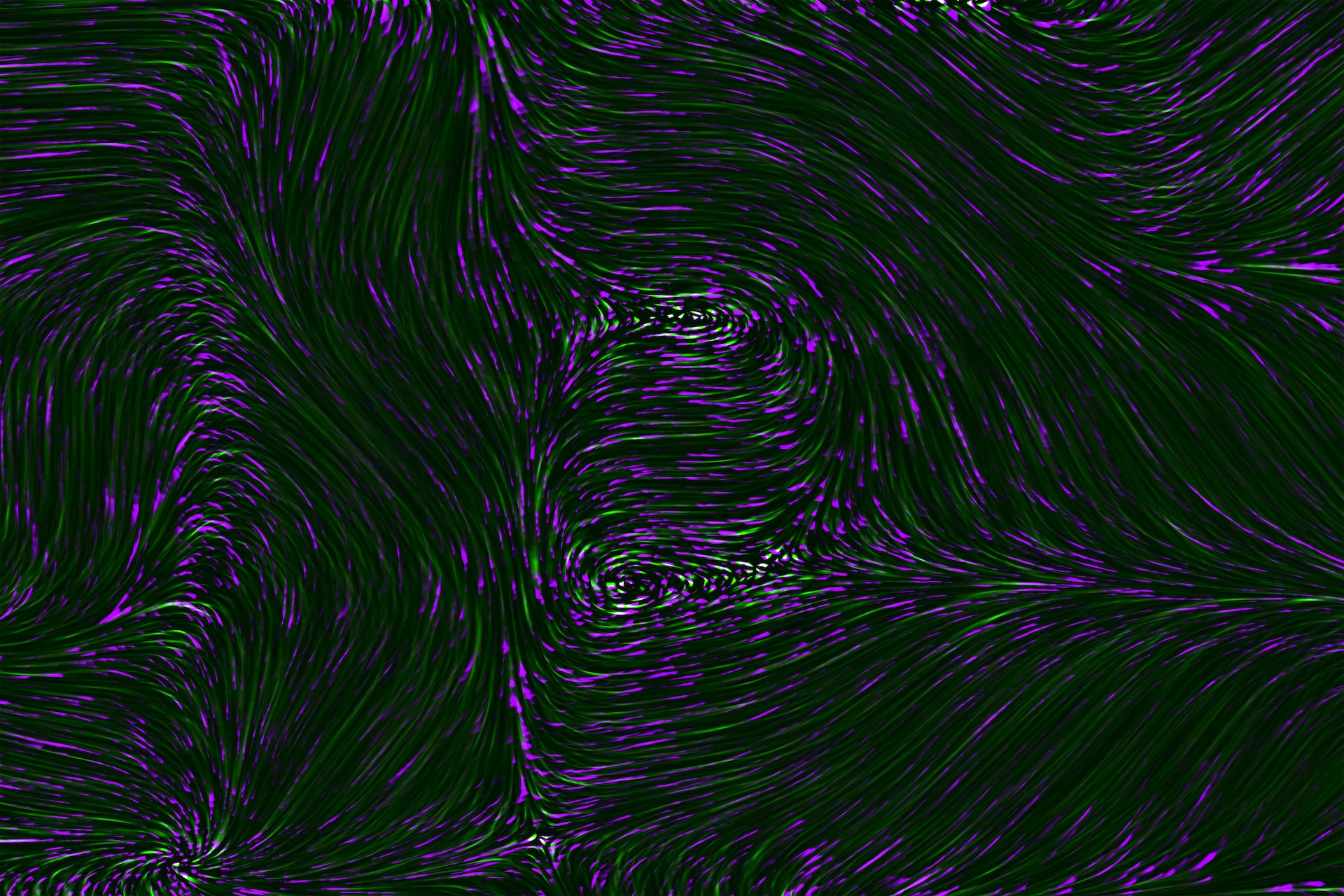
Contact US
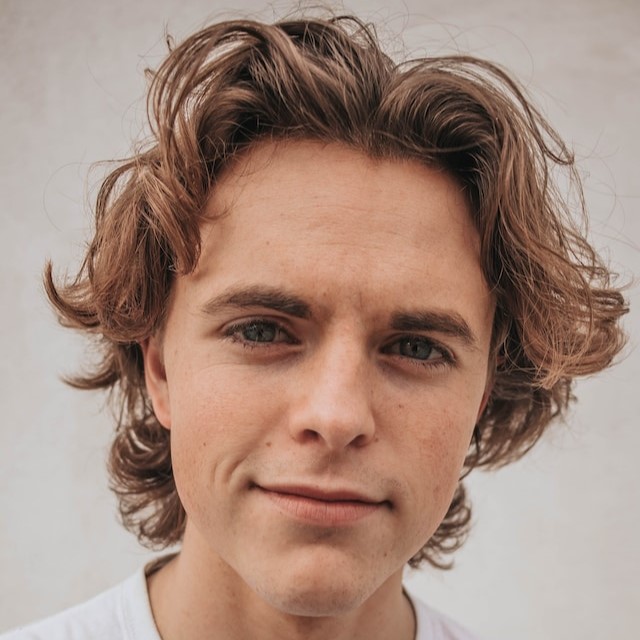
James V.
Company CEO